How the Cable Industry Is Benefiting from Machine Learning (and We’re Just Getting Started)
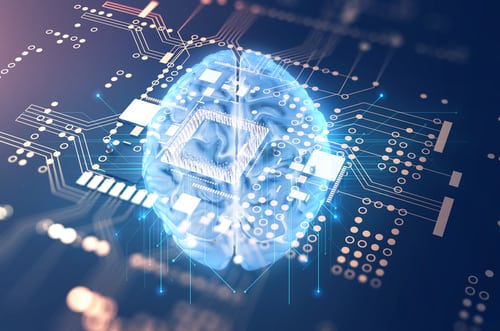
By Chris Bastian
Senior Vice President, Engineering & CTO
SCTE-ISBE
Cable network operators are more and more embracing the advantages that artificial intelligence (AI) and machine learning (ML) can bring to all phases of running their networks, including network architecture, design, engineering, and operations. In many scenarios, AI and ML computing algorithms are complementing human efforts to optimize network operation by analyzing multiple sources of data, making actionable recommendations, and oftentimes correcting network impairments before customers even notice them.
First, a few words about terminology. Artificial intelligence and machine learning are often used interchangeably; however, there are distinct differences.
Artificial intelligence is a slightly older term, attributed to a team at Dartmouth College in 1956, and is a branch of analytics where the computing system is able to reason without human intervention. Examples of AI are speech and image recognition.
Machine learning is a subset of artificial intelligence and also dates back to the 1950s, although it wasn’t until the 1980s that the term became widely used. ML is the ability to automatically learn and improve from experience without being explicitly programmed. From Nvidia, “Rather than hand-coding software routines with a specific set of instructions to accomplish a particular task, the machine is ‘trained’ using large amounts of data and algorithms that give it the ability to learn how to perform the task.” An ML example is Google Maps recommending the best way to not only reach your destination but even where to park, based on all the traffic and associated data that Google compiles.
Other industries such as manufacturing, transportation, finance, and education are applying machine learning to improve both their operations as well as customer experience. One example in the finance world is the “robo-advisor,” which uses ML algorithms to build a financial portfolio that ideally matches the goals and risk tolerances identified by the individual user.
As indicated, the cable industry is also in the midst of applying ML to many of the challenges we face in day-to-day operations. While visiting with network operators, I am seeing many applied examples of machine learning, and many of these case studies are now being shared in technical papers and public forums for the benefit of the overall industry.
One cable operator example is applying machine learning to HFC node splits. Node splits are a common tool in the network operator’s toolkit, with the goal to provide more bandwidth and capacity to a given geographic area. Node splits can involve both “virtual” and “physical” means. Virtual splits are assigning lasers to nodes on a one-to-one basis, while in the case of physical splits, two or more nodes are deployed where one node had served before. Physical splits may also coincide with driving fiber deeper into a geographic area. Both types of node splits require labor and capital investment, so the cable operator needs to prioritize where to spend its limited financial resources. Historically, this prioritization has required an intensive manual effort. This is where machine learning comes in. The node split decision algorithm considers multiple variables, including service load and cost, and this cable operator is harnessing ML to analyze all of these variables and provide an actionable and prioritized split report.
Another cable operator example is applying machine learning to spectral impairment detection in the access network. Machine learning trained numerous sets of labeled spectral impairment observations and used this method to build a model for impairment detection, including 15 different types of impairment such as tilt, roll-off, notch, and foreign carrier. This ML method returns a complete impairment diagnostic including all impairment instances detected on a spectral signal.
We are only starting to scratch the surface where AI and ML can lend a hand in building and operating more complex and robust networks and services. Over the next few years, machine learning will help optimize network operations in hundreds if not thousands of cases.
SCTE•ISBE Cable Tec Expo® 2018, conducted in Atlanta Oct. 22–25, featured several papers and presentations on AI and ML from CableLabs®, Comcast, Guavus, Intel, Accenture, and others. Download them at https://www.onlineevent.com/scte-isbe/events/1309 and hold the dates for next year’s Expo—Monday through Thursday, Sept. 30–Oct. 3, in New Orleans.